Data Centric Research from a Home Office
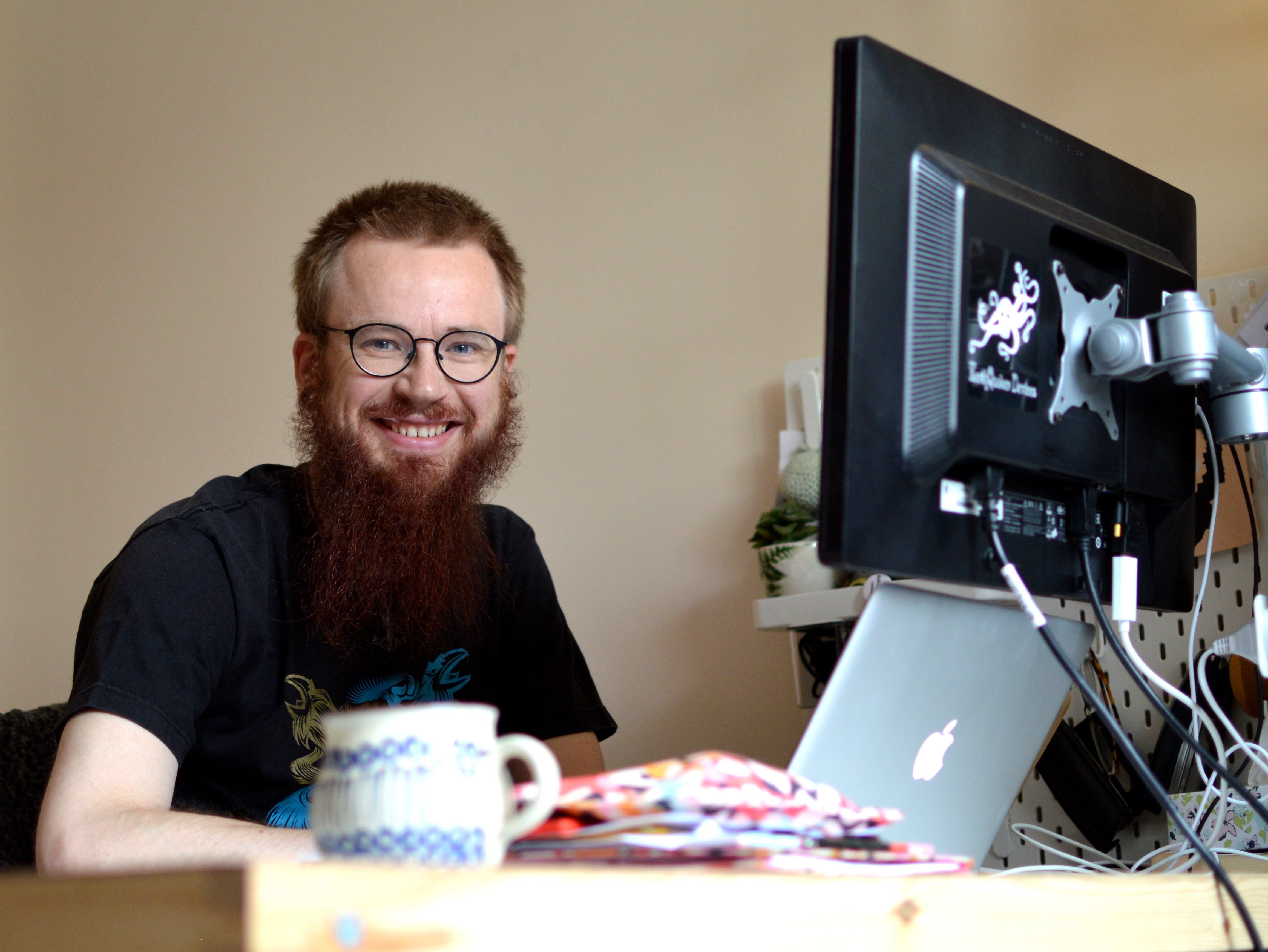
Author: Mikkel Bue Lykkegaard
It has been an interesting year, to put it mildly. When the first lockdown struck, I was officially 6 months into my PhD, but had been working on it for a while longer. Since my work is mostly taking place on a computer, it did not affect me as much as some of my peers. I dug into my numerical experiments from my bedroom-come-home-office, and video meetings became the modus operandi of sharing ideas, challenges, and general workplace banter.
It has now been over a year since the first lockdown, and a highly productive one, in spite of the disruption. We had our Multilevel Delayed Acceptance (MLDA) sampler accepted into the upstream PyMC3 code and contributed a tutorial to the PyMCon 2020 conference. We also participated in the Machine Learning for Engineering Modeling, Simulation, and Design workshop at NeurIPS 2020 with a conference paper on the same topic.
On the more applied side of things, I had my first paper published in Computer Methods in Applied Mechanics and Engineering (CMAME) after a fairly long review process. It was not that there were that many suggested changes, but I think the reviewers, like everyone else, had unexpected challenges to deal with, aside from reviewing my research. In the paper, we develop a Metropolis-Hastings algorithm that exploits a Deep Neural Network proxy to accelerate uncertainty quantification of groundwater flow. The reviewers had mostly nice things to say about it, so I am really happy to have made my first, but hopefully not last, contribution to science. I will be presenting this research at the Digital World Water Conference, which was originally meant to be a traditional physical conference but was moved online for obvious reasons. Anyway, the PhD is not a time to rest on my laurels (at least not for too long), so onwards an upwards.
Our next project is about developing a methodology for adaptive optimal design of groundwater surveys. For the uninitiated, this translates roughly into “Where to Drill Next?”, which is one of the original motivations for my PhD. It (unsurprisingly) turns out that the answer to that question is less than obvious. Looking for an optimal place to take a groundwater sample begs the question "optimal with respect to what?", which is completely problem-dependent, and the numerics are non-trivial. While not universally applicable, we have developed a methodology that exploits the adjoint of our stochastic groundwater model to explore uncertain model sensitivities throughout our groundwater modeling domain. The details are fairly technical and there are still some unanswered questions, but I am running the experiments as I write this, and a publication will follow as soon as we have worked out the details.
All things considered, it has been a pretty good year. Our research group has been supportive all the way through, and while certain aspects of academic life have been reduced (such as going to actual, physical conferences and the obligatory pint-after-work), unexpected opportunities have presented themselves from the vacuum. I think - hope - that looking back on this unusual time, we can perceive it, not as a period of restriction, but as one of discovery.

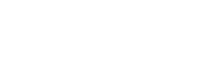