Project Bluebird: An AI system for air traffic control

Start Date 01/07/21 - Finish Date 30/06/26
Funding. EPSRC Prosperity Partnership 5 Year, 13.75M
Advancing probabilistic machine learning to deliver safer, more efficient, and predictable air traffic control
Air traffic control (ATC) is a remarkably complex task. In the UK alone, air traffic controllers handle as many as 8,000 planes per day, issuing instructions to keep aircraft safely separated. Although the aviation industry has been hit by the pandemic, European air traffic is forecast to return to pre-pandemic levels within five years. In the long term, rising passenger numbers and the proliferation of uncrewed aircraft will mean that UK airspace is busier than ever, so next-generation ATC systems are needed to choreograph plane movements as efficiently as possible, keeping our skies safe while reducing fuel burn.
The project has three main research themes:
Develop a probabilistic digital twin of UK airspace. This real-time, physics-based computer model will predict future flight trajectories and their likelihoods – essential information for decision-making. It will be trained on a NATS dataset of at least 10 million flight records, and will take into account the many uncertainties in ATC, such as weather, or aircraft performance.
Build a machine learning system that collaborates with humans to control UK airspace. Unlike current human-centric approaches, this system will simultaneously focus on both the immediate, high-risk detection of potential aircraft conflicts, and the lower risk strategic planning of the entire airspace, thus increasing the efficiency of ATC decision-making. To achieve this, researchers will develop algorithms that use the latest machine learning techniques, such as reinforcement learning, to optimise aircraft paths.
Design methods and tools that promote safe, explainable and trustworthy use of AI in air traffic control systems. This will involve experiments with controllers to understand how they make decisions, so that these behaviours can be taught to AI systems. The project will also explore ethical questions such as where the responsibility lies if a human-AI system makes a mistake, how to build a system that is trusted by humans, and how to balance the need for both safety and efficiency.
Recent updates
We will be recruiting for additional team members as the project progresses, and will link to the opportunities below as they arise.
Research Associate, Probabilistic Machine Learning x2 closes 5 September 23:59
Research Associate, Machine Learning Control closes 5 September 23:59
Exeter / Turing Research Fellow in Machine Learning Control closes 5 September 23:59
Exeter / Turing Research Fellow in Human-Computer Interaction closes 5 September 23:59
Exeter / Turing Research Fellow in Probabilistic Machine Learning closes 5 September 23:59
Collaborators
Professor Mark Girolami, https://www.turing.ac.uk/people/researchers/mark-girolami
Professor Richard Everson, https://emps.exeter.ac.uk/computer-science/staff/reverson
Dr. Adrian Weller, https://www.turing.ac.uk/people/researchers/adrian-weller
Dr. Edmond Awad,https://business-school.exeter.ac.uk/about/people/profile/index.php?web_id=Edmond_Awad
Dr. Evelina Gabasova, https://www.turing.ac.uk/people/researchers/evelina-gabasova

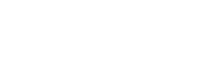