
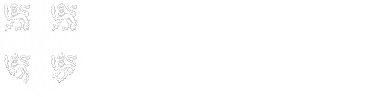

Intelligent Virtual Test Pyramids for High Value Manufacturing

Start Date 01/10/19 - Finish Date 30/09/24
Funding. UKRI 5 Year Turing AI Fellowship ∼£2.6M
Developing novel AI methods to build a more sustainable aviation industry
There is a paradox in aerospace manufacturing. The aim is to design an aircraft that has a very small probability of failing. Yet to remain commercially viable, a manufacturer can afford only a few tests of the fully assembled plane. How can engineers confidently predict the predict the failure of a low-probability event? This research is developing novel, unified AI methods to intelligently fuses models and data, enabling industry to slash conservatism in engineering design, leading to faster, lighter, more sustainable aircraft.
The engineering solution to building a safe aircraft is the test pyramid. Variability in structural performance is quantified from thousands of small tests of the material (a coupon or element test), hundreds of tests at the intermediate length scales (called details components) and a handful of tests of the full system. Sequentially, the lower length scales derive ‘design allowables’ for tests at the higher levels. Yet, such derivation is accompanied by significant uncertainty.
In practice, this uncertainty means that ad hoc ‘engineering safety factors’ have to be applied at all length scales. As we seek a more sustainable aviation industry, the compound effect of these safety factors leads to significant overdesign of structures. The overdesign results in extra weight, which severely limits the efficiency of modern aircraft. A clear route to flying lighter, more efficient aircraft is to better quantify the uncertainties inherent in the engineering test pyramid, which will allow the industry to confidently trim excessive safety factors.
New high-fidelity simulation capabilities in composites now mean that mathematical models can supplement limited experimental data at the higher length scales. Yet, with this comes additional uncertainties from the models themselves. Existing experimental tests, particularly at the lower length scales, can be used to reduce and quantify uncertainty in the models. The typical typical statistical approach is Bayesian, in which the distribution of a model?s parameters is learned to fit the data probabilistically. However, existing capabilities are notoriously computationally expensive, often limited to small-scale applications and simplified experimental data sets. For real engineering test pyramids, the available data and models are more heterogeneous and their connections are are complex, driving the need for new fundamental research.
This research will develop a novel, unified AI framework that intelligently fuses models and data at all levels of the engineering test pyramid, remains computationally feasible and is sufficiently robust to support ethical engineering decision-making in order to slash conservatism in engineering design, leading to faster, lighter, more sustainable aircraft.
Recent Updates
- Michael Gibson and Ben Fourcin, started 6 July 2020.
- With City Science we have reviewed available modelling software for engineering consumption, and have down selected “EnergyPlus”.
- Obtain building information data for Living Systems Institute (LSI)
Collaborators
- Professor Tim Dodwell, Academic Principle Investigator, https://emps.exeter.ac.uk/engineering/staff/td336
- Professor Mark Girolami, http://www.eng.cam.ac.uk/profiles/mag92
- Professor Philip Withers, https://www.royce.ac.uk/about-us/professor-phil-withers/
Partners

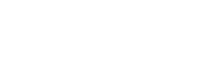