Multi-Level Inversion and Uncertainty Quantification for Groundwater Flow and Transport Modelling
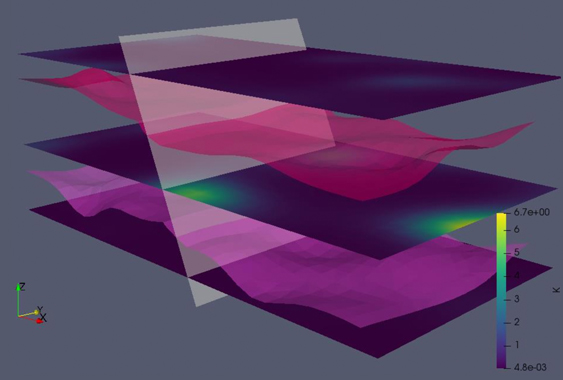
Start Date 24/09/18 - Finish Date 23/09/22
Investigating cutting edge multi-level Markov Chain Monte Carlo methods and machine learning techniques to improve inversion and uncertainty quantification in hydrogeology
The problem being addressed is the prohibitive computational cost of doing standard MCMC on high-dimensional distributions with expensive forwards models, such as groundwater flow and transport problems. One solution is the use of surrogate models in multi-level MCMC model hierarchies, and we are currently exploring various machine learning techniques for ultra-fast approximation of model response. The work has applications in both groundwater abstraction and remediation – improved estimates of groundwater flow patterns can improve decision support systems, allowing groundwater abstraction companies to make better sustainable yield estimates, and remediation companies to design taylor-made remediation campaigns.
Inversion of distributed environmental models is typically an ill-posed problem with noisy data. This is a well known challenge that has been addressed from various angles throughout the previous half century. Markov Chain Monte Carlo (MCMC) overcomes the ill-posedness by treating models as realisations of a stochastic process, allowing for rigorous uncertainty quantification. However, this power oftentimes comes at a prohibitive computational cost, since MCMC requires the forward model to be evaluated many times and since, in case of groundwater flow and potentially an advection/diffusion process, forward model evaluation involves solving a Partial Differential Equation (PDE).
Delayed Acceptance or Multi-Level MCMC alleviates some of the model evaluation cost by introducing a model hierarchy, in which an inexpensive reduced-order or surrogate model is employed as a filter by rejecting highly unlikely parameter sets. Hence, a carefully chosen reduced-order model can accelerate MCMC significantly. Emerging machine learning techniques provide ultra-fast predictions for non-linear problems if tuned correctly and may thus deliver previously unimaginable improvements to multi-level MCMC.
This project is about identifying promising reduced-order models and multi-level MCMC techniques that would allow fast inversion and uncertainty quantification of hydrogeological problems.
Recent Updates
We are currently working on a journal paper describing the use of a Deep Neural Network as a surrogate model. The underlying code makes use of the high-performance PDE solver FEniCS along with the popular neural network framework Keras and an in-house MCMC code.
Collaborators
- Professor Tim Dodwell, Academic Principle Investigator, https://emps.exeter.ac.uk/engineering/staff/td336
- Dr David Moxey, PhD Supervisor, https://emps.exeter.ac.uk/engineering/staff/dm550

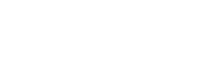